Blackbox Control Design for inaccurate Models
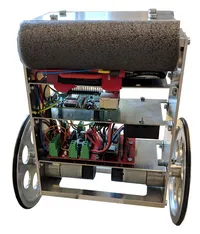
When an analytic control design is difficult, e.g. because of very nonlinear model, alternative methods from the field of machine learning can be used, e.g. policy gradient methods, gradient free optimization or approximate explicit MPC. Those methods provide a feedback controller in the form of a parametric function, with the behavior being defined in a cost function. Since the controller is trained in simulation on a simplified model, the application of the control on the real system can show significantly worse performance, depending on the accuracy of the simulation model. This loss in performance can be reduced by introducing changes in the control design phase, and the derivation and testing of those methods is part of the research at our chair.
Person in charge: Christian Dengler, M. Sc., PhD at the chair from 2015 to 2020