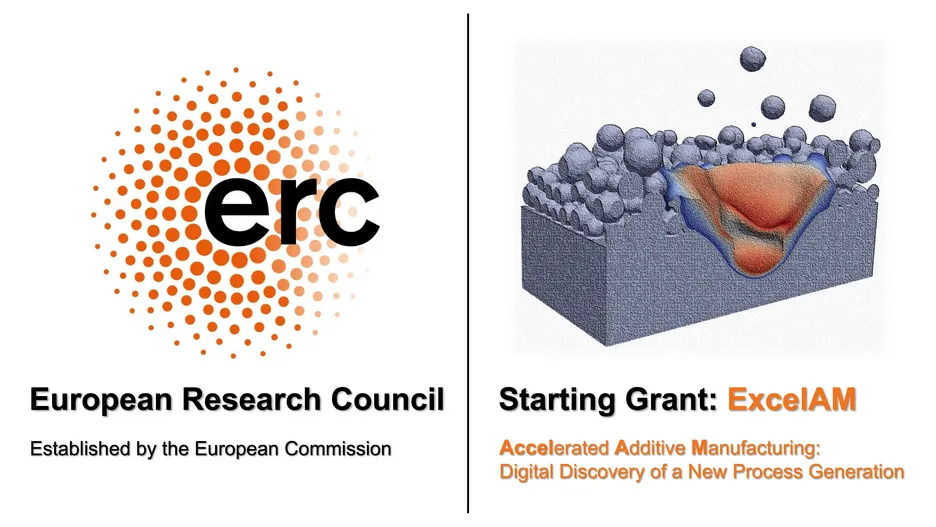
Additive Manufacturing (AM) by Laser Powder Bed Fusion (LPBF) has the potential to revolutionize future product development, design and supply chains. Since the underlying multi-scale physics are not sufficiently understood, its potential cannot be fully exploited. Sub-optimal process conditions lead to severe defects on different scales, rendering parts unsuitable for use. Critically, known regimes of stable processing go along with very low built rates, i.e., very high costs compared to other processes. This limits LPBF to high value applications such as medical devices but prohibits applications in mass production, where it otherwise could allow for entirely new technologies.
The ERC Starting Grant project ExcelAM aims at the digital discovery of new high-throughput process regimes in LPBF, to increase build rates by at least one order of magnitude. For this purpose, novel computational models are developed and employed, unifying innovative aspects of high-fidelity multi-physics modelling, data-based approaches and high-performance computing. Based on these cutting-edge tools, ExcelAM aims to push the limits of LPBF from pure high value applications towards mass production. Moreover, by making them available to the public, ExcelAM will help scientists and practitioners in the field of production engineering and beyond to face the technological challenges of the 21st century.
The research concept of ExcelAM consists of five main work packages: These contain the development of novel mesoscale models for highly cohesive metal powders, for the complex melt pool dynamics in LPBF including non-equilibrium evaporation phenomena, and for the resulting coupled melt-vapor/gas-powder dynamics (WP1 and WP2). A further focus lies on the development of high-performance computing models for thermo-mechanical and microstructure predictions on the scale of realistic part sizes (WP3). Modern Bayesian learning schemes will be employed for inverse identification of the underlying model and material parameters, which are typically very challenging to obtain by conventional means of material characterization, given the extreme process conditions prevalent in LPBF (WP4). Eventually, these computational modeling approaches on different length scales will be combined for a simulation-based exploration of novel process strategies and physical regimes in LPBF that allow for a significantly increased production throughput (WP5). In this project phase, first steps towards a practical implementation of the most promising concepts will be undertaken, exploiting custom-build LPBF testbeds of our close collaborators, e.g., the MIT Mechanosynthesis Group.