Research Mission
Additive Manufacturing (AM) processes such as Laser Powder Bed Fusion (LPBF) of metals have the potential to revolutionize product development and design as well as the structure of future supply chains. However, since the underlying physics are not well understood, their potential can not be fully exploited at presence. Sub-optimal process conditions lead to severe defects on different scales, often rendering parts unsuitable for use. Critically, known regimes of stable processing often go along with low built rates, i.e., high costs compared to other processes. This limits LPBF to selected high value applications such as medical devices but prohibits applications in mass production where it otherwise could allow for new technological solutions.
Through high-fidelity computational models, we aim to gain fundamental understanding of the underlying physics and, eventually, to inspire new process strategies and to identify new physical regimes, overcoming the limitations of existing processes. As an example, the ERC Starting Grant ExcelAM focusses on the digital discovery of new high throughput process strategies in laser powder bed fusion. It aims to push the limits of LPBF from pure high value applications towards mass production.
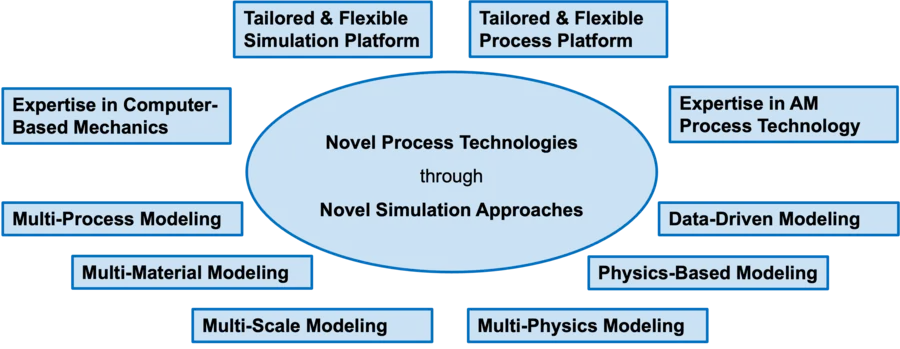
In short, our research mission might be stated as “Novel Process Technologies through Novel Simulation Approaches”. We believe that a truly predictive, simulation-based exploration of novel process technologies requires a wholistic modeling approach that covers the most relevant physics and length-scales of the process, or in other words: a multi-physics and multi-scale modeling approach. It equally incorporates aspects of physics-based and data-driven modeling. We aim at first-principle modeling approaches of high generality, i.e., with a minimum of process-specific assumptions. While our research has a focus on laser powder bed fusion of metals, this first-principle modeling approach allows for a very good transferability to other processes and material classes, or in short: for multi-material and multi-process modeling. For example, in our recent contribution Fuchs et al. 2022 the transferability of a computational framework for multi-phase flows from Laser Powder Bed Fusion (LPBF) to other AM processes such as Binder Jetting (BJ), Directed Energy Deposition (DED) and Material Jetting (MJ) has been successfully demonstrated. To put this research philosophy into practice, we rely on a strong expertise in computer-based mechanics covering aspects of computational solid, structural, fluid and granular mechanics.
In additive manufacturing of metals, we particularly focus on the modeling of cohesive metal powders, complex multiphase flows with phase change, thermo-solid-mechanics on part-scale, and the metallurgical microstructure evolution during the process. Moreover, our research incorporates a broad spectrum of discretization schemes such as the finite element method (FEM), the discontinuous Galerkin (DG) method, the discrete element method (DEM), and smoothed particle hydrodynamics (SPH) as well as CutFEM and CutDG approaches. Also enabling technologies for large-scale engineering simulations, i.e., numerical solution schemes, scalable algorithms, parallel software development, and high-performance computing are part of our research. In particular, it is essential for our research to develop our own simulation tools. Only with a tailored and fully flexible simulation platform, which allows to adapt every aspect of the underlying models, numerical schemes and algorithms, the required accuracy, robustness and efficiency can be achieved to allow for meaningful simulations of AM processes on practically relevant length and time scales. Among others, we are one of the main contributors to the recently released open-source research code 4C (https://github.com/4C-multiphysics/4C). On the process side, we rely on tailored and flexible experimental AM platforms of our collaborators (e.g., the MIT Mechanosynthesis Group) to implement new simulation-inspired process strategies, which might exceed the capabilities of commercially available AM hardware.