Bayesian Modeling & Active Learning
Quantifying the uncertainty of molecular models and the posterior robust prediction of simulation results. Using uncertainty to build informative training datasets step by step in an active learning loop.
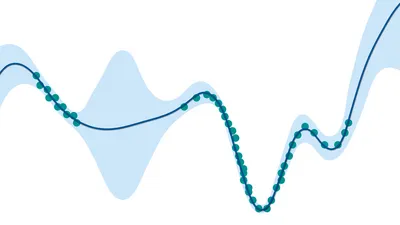
Recent Papers:
Active learning graph neural networks for partial charge prediction of metal-organic frameworks via dropout Monte Carlo
S. Thaler, F. Mayer, T. Siby, A. Gagliardi, J. Zavadlav, Npj Comput. Mater. 2024, paper, GitHub
Partial charge prediction for Metal Organic Frameworks. The Dropout Monte Carlo is computationally cheaper than the conventional ensemble approach. At the same time, it provides a reliable estimation of the mean absolute error for unseen MOFs, enabling an efficient active learning strategy.