Machine Learning Augmented Molecular Simulations
Advancing model fidelity with multi-body potentials parametrized by deep learning architectures and learning dynamics of molecular systems.
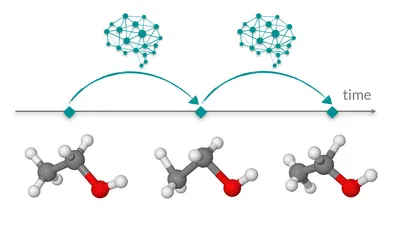
Recent Papers:
Predicting solvation free energies with an implicit solvent machine learning potential
Accurate machine learning force fields via experimental and simulation data fusion
Deep Coarse-grained Potentials via Relative Entropy Minimization
S. Thaler, M. Stupp, J. Zavadlav, J. Chem. Phys. 2022, paper, arXiv, GitHub
Coarse-grained ML potentials trained with Relative Entropy result in more acurate potential energy srufaces, require less data and can be employed with larger integration timesteps compared to the conventional training with Force Matching.
Learning neural network potentials from experimental data via Differentiable Trajectory Reweighting
S. Thaler, J. Zavadlav, Nat. Commun. 2021, paper, arXiv, GitHub
received MDSI Best Paper of Year 2021 Award
selected as Editors’ Highlights paper in Applied physics and mathematics research, featured in Nat. Commun. collection Molecular Dynamics simulations and Computational methods in Life Science.
Efficient top-down parametrization of deep neural network molecular models, enabling molecular models to exactly match experimental observations.
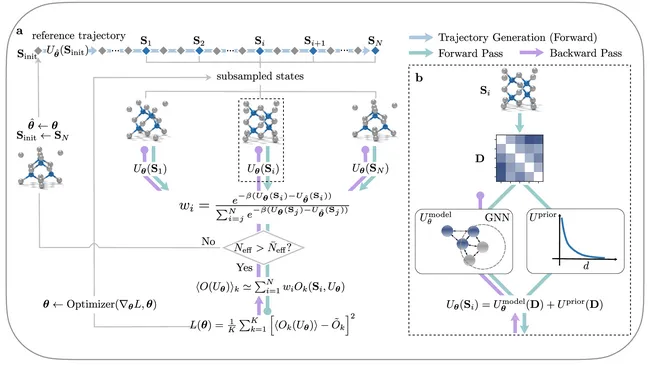